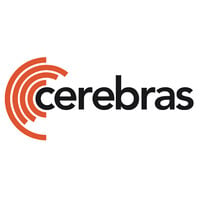
Cerebras Systems
Similar Companies Hiring
Jobs at Cerebras Systems
Let Your Resume Do The Work
Upload your resume to be matched with jobs you’re a great fit for.
Success! We’ll use this to further personalize your experience.
Recently posted jobs
Artificial Intelligence
The Head of IT will oversee IT systems to enhance employee experience, manage corporate IT services and enterprise platforms, and lead IT teams to align technology with business goals.
Artificial Intelligence
Lead the definition and design of storage solutions for AI and HPC cluster deployments, ensuring alignment with performance and security standards through vendor evaluations and internal configurations.
Artificial Intelligence
The role involves automating cluster configurations, developing scheduling systems, monitoring resource failures, and creating user and admin tools for large-scale AI supercomputers.
Artificial Intelligence
The role involves integrating software and hardware for Cerebras' AI platform, focusing on quality assurance, debugging, and testing methodologies. Responsibilities include automating workflows and collaborating with teams to ensure product quality and performance.
Artificial Intelligence
Responsible for optimizing AI cloud platform deployment, ensuring high reliability and scalability of distributed training and inference infrastructure, and developing tools to identify system bottlenecks.
Artificial Intelligence
Design and build responsive frontend interfaces for AI cloud products, ensuring optimal user experience and handling high traffic efficiently.
Artificial Intelligence
The role involves deploying and managing clusters in complex environments, troubleshooting issues, and collaborating with teams to ensure deployment success.
Artificial Intelligence
Manage and operate cutting-edge AI compute infrastructure clusters while ensuring performance, availability, and troubleshooting complex systems. Drive optimization and contribute to enhancements in monitoring processes.
Artificial Intelligence
Innovate and execute tests on AI infrastructure, developing optimized test strategies, and ensuring automated tests for efficiency and scalability across complex distributed systems.
Artificial Intelligence
Assist in automating and supporting large-scale AI cluster operations, contribute to orchestration systems, and develop monitoring and job management tools.
Artificial Intelligence
Lead the design and buildout of cybersecurity detection and response capabilities, oversee a SOC, and enhance automation for incident response.
Artificial Intelligence
The Product Manager Intern will design and implement a web-based configuration tool for HPC appliances, working on both front-end and back-end development while collaborating with technical teams to understand user requirements.
Artificial Intelligence
As an AI Cloud Platform Software Engineer, you will optimize cloud services for AI training and inference, ensuring reliability and efficiency while addressing system bottlenecks.
Artificial Intelligence
Cerebras seeks a Senior Runtime Performance Engineer to optimize AI applications on x86 architecture, focusing on CPU, memory, and performance profiling.
Artificial Intelligence
As a Senior ML Quality Engineer, you will ensure software quality across ML workloads, focusing on testing, validation, automation, and improving debugging methodologies.
Artificial Intelligence
The Senior ML Frameworks Engineer designs APIs for integrating ML frameworks with Cerebras' architecture, enhancing performance and ease of use while leading ML integration projects.
Artificial Intelligence
Build performance models, optimize Kernel micro code, debug runtime performance, design performance features, and develop tools for performance data visualization.
Artificial Intelligence
The role involves designing and expanding build tool functionality, debugging ML application issues, and collaborating with teams to enhance Cerebras' solutions in AI.
Artificial Intelligence
The role involves designing and expanding build tool functionality, debugging ML applications, and collaborating with cross-functional teams to enhance leadership in AI solutions.
Artificial Intelligence
The role involves integrating and quality assurance of software and hardware for Cerebras AI platform, focusing on automation, debugging, collaboration, and quality metrics delivery.